Artificial Intelligence (AI) and Machine Learning (ML) in Discrepancy Management (Clinical Data Management)
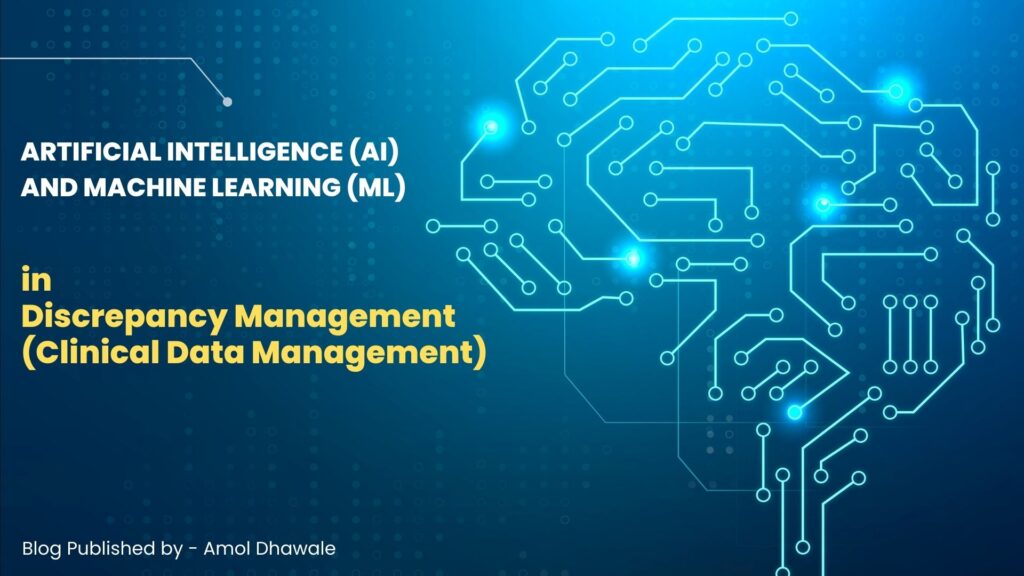
Pharma companies conducting their own trials are always investing more in new ideas and innovations just to reduce the time span that is required to bring any drug molecule to the market. In last one decade we have seen tremendous changes in the way of clinical trials conduct. Global regulatory authorities are also supporting new way of clinical trials and allowing necessary amendments to their rules and regulations.
Electronic technologies have increased ease of data availability, data usage for predictive and prescriptive data analysis and data analytics.
eCRFs consists of FEC (Field Edit Checks), Edit checks and CF (Custom Functions). However, it is not possible to find out all types of the discrepant data through these eCRFs capabilities. Hence, human intelligence is required to perform various complicated data analysis and data review activities which is done through manual data review or analysis activity.
Considering AI and ML capabilities, it seems possible that the human intelligence could be replaced by AI or AI could help to have minimal human intervention for performing manual data review and frame queries. Redundant and or repetitive activities like query drafting and raising could be automated using ML
- Can AI/ML replace manual data review activities that are being done by data managers?
- Can AI/ML replace repetitive or monotonous activity like query drafting and raising on eCRFs ?
With respect to study conduct activities, data managers and site monitors or CRA need to invest their 75% of daily routine time in manual data review and discrepancy management. Tools like J-review and SAS listings are being used to find the discrepant data and data managers need to frame manual queries and raise it on appropriate eCRF and data fields. Various complicated checks could not be run using tools and hence, data managers are manually doing the review using MS Excel and then frame, raise queries on eCRFs
Use of AI and ML in discrepancy management process could reduce workload for data managers and site monitors (up to some extent). Data managers will be able to work on more studies at a time and this will have positive impact on ROI for sponsor companies. Due to anticipated less efforts by the data managers, study budget could be reduced for data management. Human errors could be avoided through use of automation and ultimately quality data will be available at the study database lock.
1. Introduction:
Every year number of trials are initiated and or run by top pharmaceutical companies. Hardly very few trials are completed on time as per project plan. Due to COVID-19 pandemic spread, world has understood value and importance of clinical trials and impacts of not having drug in hand on time when it is really required. Due to uncontrolled spread of corona virus, healthcare professionals and every individual who is part of drug discovery and drug development are playing their role and trying level best not only to invent a new drug but to save mankind.
Pharma companies conducting their own trials are always investing more in new ideas and innovations just to reduce the time span that is required to bring any drug molecule to the market. In last one decade we have seen tremendous changes in the way of clinical trials conduct. Global regulatory authorities are also supporting new way of clinical trials and allowing necessary amendments to their rules and regulations.
Electronic technologies have increased ease of data availability, data usage for predictive and prescriptive data analysis and data analytics.
All the phases included in drug discovery and drug development are important and critical; however, when drug under investigation enters in human body, the crucial and most important phase starts i.e. clinical trials. “DATA” is common thread, which connects one step to another with the help of positive treatment results. All types of results (positive and negative) are altogether collected in Clinical Data Management System (CDMS) and used for analysis. eCRFs are used to collect patient data or subject data for various phases of trials i.e. I, II, III. Clinical data managers are the one who designs, maintains and freeze the eCRFs. Data management has unique workflow which basically includes study start-up or study design, study conduct and study close-out activities.
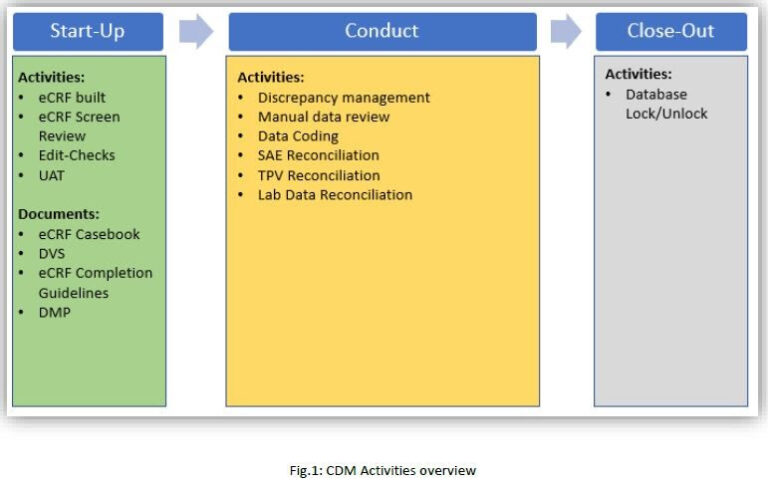
1.1 Purpose
eCRFs consists of FEC (Field Edit Checks), Edit checks and CF (Custom Functions). However, it is not possible to find out all types of the discrepant data through these eCRFs capabilities. Hence, human intelligence is required to perform various complicated data analysis and data review activities which is done through manual data review or analysis activity.
Considering AI and ML capabilities, it seems possible that the human intelligence could be replaced by AI or AI could help to have minimal human intervention for performing manual data review and frame queries. Redundant and or repetitive activities like query drafting and raising could be automated using ML
Methods:
➢ Can AI/ML replace manual data review activities that are being done by data managers?
➢ Can AI/ML replace repetitive or monotonous activity like query drafting and raising on eCRFs ?
2.1 Background:
– With respect to study conduct activities, data managers and site monitors or CRA need to invest their 75% of daily routine time in manual data review and discrepancy management.
– Tools like J-review and SAS listings are being used to find the discrepant data and data managers need to frame manual queries and raise it on appropriate eCRF and data fields.
– Various complicated checks could not be run using tools and hence, data managers are manually doing the review using MS Excel and then frame, raise queries on eCRFs.
2.2 Existing EDC data collection method:
Initially site used to have pCRFs i.e. CRFs in paper format and record the patient data on paper CRFs with pen. Having pCRFS for the study was time consuming and lengthy process. Gradually, pCRFs were replaced by EDC and trial stakeholders are now able to view real time data. Existing EDC data collection method includes but not limited to below points:
1. As per study protocol, eCRFs are designed, tested and ready for the study conduct activities using EDC platforms like Medidata RAVE, PhaseForward Inform etc. Edit-checks are built to have continuous automated data cleaning process in place.
2. Using data reconciliation process, EDC data is reconciled with data from other sources e.g. Patient Safety data, Laboratory data, TPV data etc. These reconciliations are performed manually i.e. data extraction from various sources, manual data review for discrepant data, raise manual queries in EDC. Also, follow up with site CRA and site personnel to get these discrepancies closed as per requirement is the time-consuming process.
3. EDC data is considered as clean when below activities are completed for all the eCRFs:
a. Site data entry completed
b. SDV completed by CRA
c. Data review completed by data manager
d. Coding completed by Medical Coder
e. All the data reconciliations are completed
f. All Open and Answered queries are closed
g. All the eCRFs are electronically signed by Principal Investigator
h. All the eCRFs are entry locked and data locked (i.e. soft lock and hard lock)
4. Data is ready for reporting and analysis at the end of data cleaning process and data managers are supposed to lock or freeze all the eCRFs of all the subjects in the study.
Commonly used eCRFs across all the therapeutic areas:
1. Subject Enrollment
2. Informed Consent
3. Date of Visit
4. Demographics
5. Vital Signs
6. Physical Examination
7. Medical History
8. Medication History
9. Inclusion/Exclusion Criteria
10. CM-Concomitant Medication
11. AE-Adverse Events
12. Drug Administration
13. Lab. Forms (Local and Central)
14. QOL – Quality OF Life Questionnaires
15. End of Study/Withdrawal
16. End of Treatment
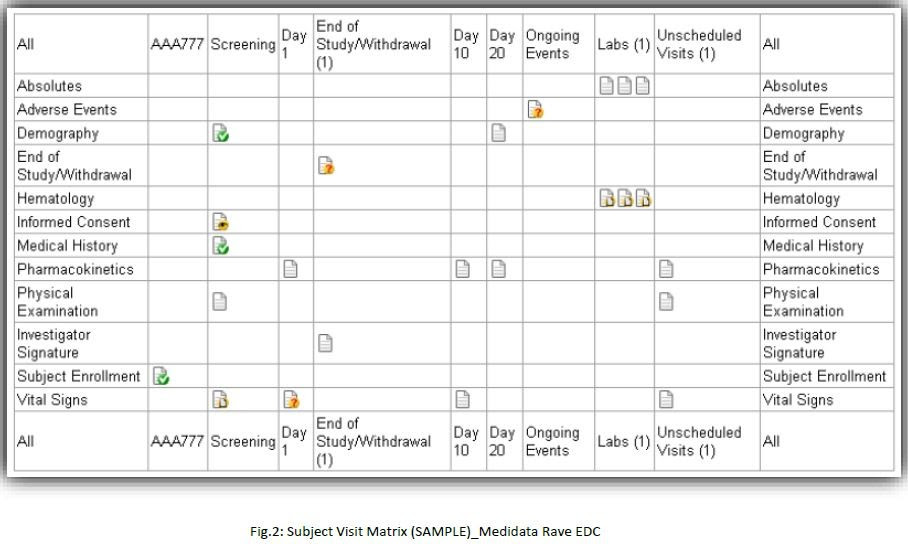
2.3 Proposal:
eCRFs consists of FEC (Field Edit Checks), Edit checks and CF (Custom Functions). However, it is not possible to find out all types of the discrepant data through these eCRFs capabilities. Hence, human intelligence is required to perform various complicated data analysis and data review activities which is done through manual data review or analysis activity.
Considering AI and ML capabilities, it seems possible that the human intelligence could be replaced by AI or AI could help to have minimal human intervention for performing manual data review and frame queries. Redundant and or repetitive activities like query drafting and raising could be automated using ML.
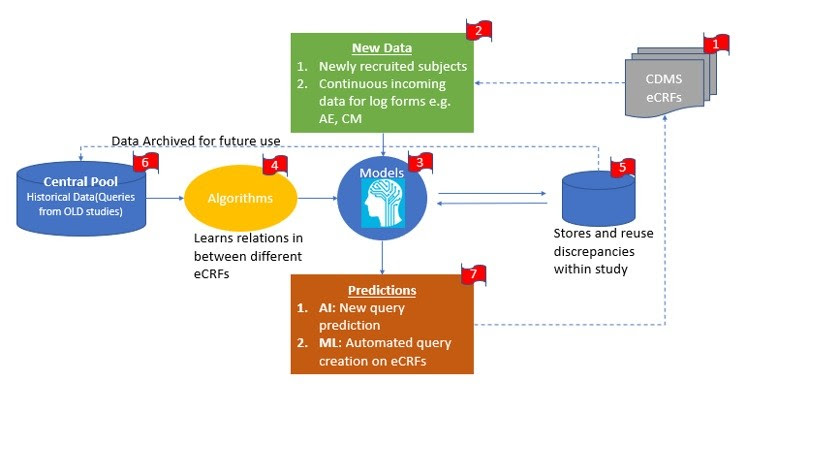
Step 1: CDMS systems like Medidata Rave, Phaseforward Inform are usually used by sponsor as a data collection tool. Site is trained to enter subject data in these system and coordinate with site monitors and clinical data managers to refine and clean the data for all the recruited subjects. Any single study contains, multiple subjects from multiple sites. Any single site contains multiple eCRF casebooks for the subjects recruited at that center or site
Step 2: Whenever new subjects is recruited, then site personnel receives new subject number through IRT system. EDC and ITR are integrated systems and hence, newly recruited subject number could be viewed in EDC.
Along with subject progress in study, site personnel enters data in EDC for subsequent study visits i.e. Screening Visit, Randomization visit and follow-up visits and so on. Log forms like Adverse Evets and Concomitant Medications can always expect data until the last study visit of the subject.
Step 3: To have automated discrepancy management process in place, system needs to develop it’s own model by learning existing process and data inputs received from site. System need to have algorithms i.e. understanding of relations in between one or more eCRFs, so that conclusions could be made and utilized to frame or draft queries. Whenever data manager enters new query with new logic, system will store this study specific new query for reuse and future references. At the same time the study specific query and query logic is stored in central pool where the pool contains all types of queries from various therapeutic areas e.g. Oncology, Cardiology, Neurology etc.
Step 4: Predictions: Considering step 1 and 2, system will be able to predict new queries and logics as per discrepant data. Based on existing discrepancies and query logic in central pool, AI can predict new queries and ML will perform repetitive task of raising similar queries to multiple forms of single subject or multiple subjects within the study.
2.4 Prototype:
This prototype depicts the automated query management system in two steps i.e. Pilot and subsequent clinical studies.
1. Pilot Clinical Studies:
Central pool and study specific pool will be empty before start of the pilot studies for any sponsor. Let’s try to compare newborn baby of man with pilot AI discrepancy management system.
Like a new AI discrepancy management system, newborn baby will not have any prior experience about anything in the life e.g. Heat is hot, ice is cold. In simple words, newborn is not aware about how to react on any condition or situation and whilst he goes through any situation for very first time, his brain understands and analyses the whole situation to take best decision and save that in memory for future references. i.e.
1. What was good and bad part
2. What was previously known information and what was completely new information.
3. What was the ideal way to react
After few years, say for e.g. when baby reaches to the age of 10 years, and then baby has number of experiences stored in his memory that could be used in his daily life. For a common child, it is expected that, his brain will utilize the past experiences to handle or to face current and future incidences in life. Obviously for an exceptional child (here, we can say AI) we can expect more.
Apart from this, brain has an ability to utilize past experiences and apply the same logic for any other future incident which has not at all faced by the individual previously. Hence, brain will interpret and predict the next best possible action to be taken and gradually it becomes habit for brain. With the help of such unconscious practice, the individual keeps growing in his life to proceed towards perfection or so called close to perfection.
Similarly, pilot AI system will take some time to learn the process, query drafting logic and store it for future use. Let’s take an e.g. of below manual check related to AE/SAE (Adverse Event / Serious Adverse Event) and CM (Concomitant Medication) module.
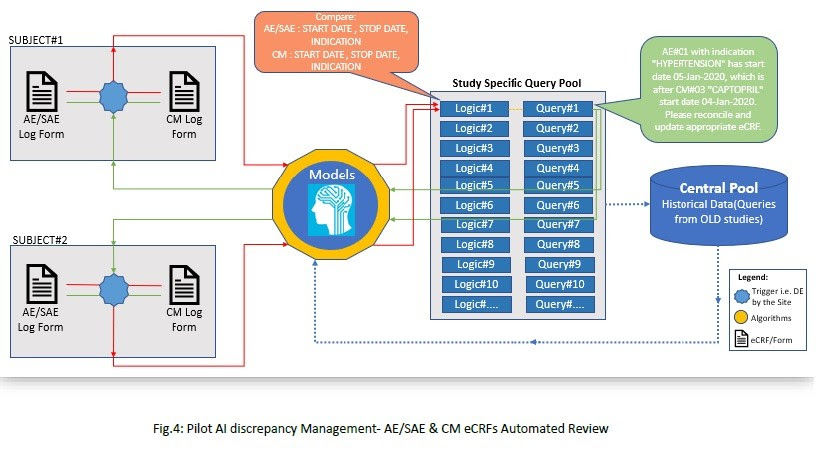
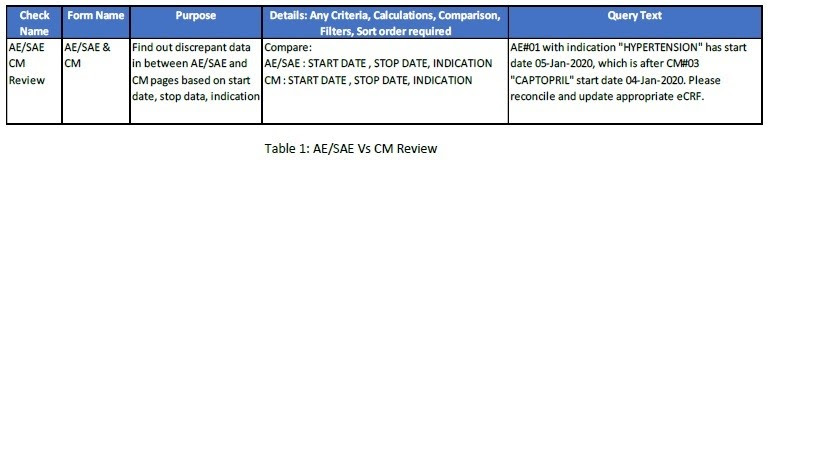
Process in Brief (Fig.4):
1. DE (Data Entry) by the investigational sites will trigger the process as and when new data
enters the CDMS.
2. Few essential points will play an important role throughout the review process are:
a. Check Name
b. Form name
c. Annotations of the fields to be compared or used
d. Additional Details: Any Criteria, Calculations, Comparison, Filters, Sort order
required
e. Query text
3. With reference to Table 1, we need to perform AE/SAE Vs CM review for subject#01 and
subjet#02 for a single clinical study. Based on the Logic#1, system will find out the
discrepant data and try to search for any available query text for the similar logic in ‘study
specific query pool’. Query#1 is already present in the system as it was raised by the data
manager.
4. Whilst subject#02 will have new data on the respective forms, then system will use the
Logic#1 and search for appropriate query text. Hence, system will be able to find query text
for this type of discrepant data i.e. Query#1. System will use the available query text by modifying AE/SAE indication, AE start and stop date as appropriate and then query will be posted on eCRFs.
5. So far, we have considered only one logic for two subjects; and multiple logics needs to be applied for all the subject’s casebook in a single study (i.e. all Study eCRFs).
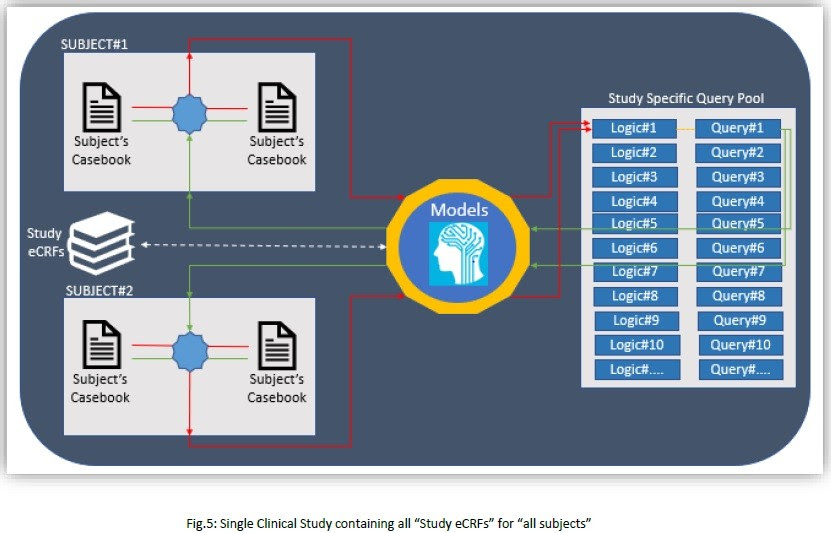
However, single pilot clinical study will not be able produce expected results and we need to proceed with more studies.
2. Subsequent Clinical Studies:
We will proceed with more studies for the automated data review process to get expected automation level. As depicted below, multiple studies will have their study specific query pool and at back end those queries will be stored at central pool; so that upcoming new studies could use appropriate historical data or queries or logics from central pool.
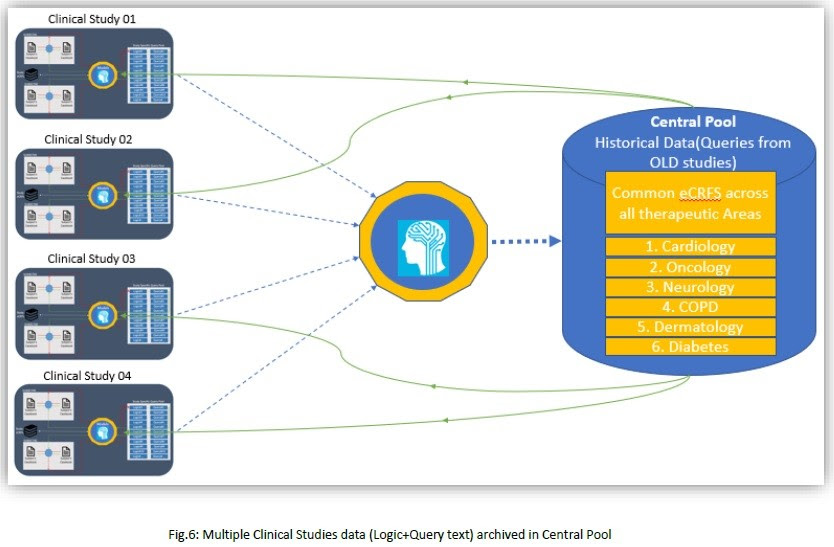
2.5 Assumptions:
1. eCRF global library will have all the common standard eCRFs used across all the therapeutic areas which will have verified eCRF with applicable data fields, edit-checks, custom functions etc. Hence, it is assumed that, the eCRF annotations will be consistent throughout all the subjects irrespective of studies.
2. This article does not focus on study specific checks automation; however, it is assumed that, system will be able to transform the traditional way of discrepancy management, and dependency upon manual review.
3. Site answered queries: System need to learn site responses provided in comment field while answering queries. However, if the data is as per expectation then system will not raise any automated queries on eCRFs.
2.6 Recommendations:
1. Use of integrated eSource platforms like ‘Clinical ink’ to collect patient source data will enhance data quality.
2. Use of integrated eCOA applications to collect trial subject’s data directly into CDMS will help to have real time clean data.
3. Results/Conclusion: Use of AI and ML in discrepancy management process could reduce workload for data managers and site monitors (up to some extent). Data managers will be able to work on more studies at a time and this will have positive impact on ROI for sponsor companies. Due to anticipated less efforts by the data managers, study budget could be reduced for data management. Human errors could be avoided through use of automation and ultimately quality data will be available at the study database lock.
5. Abbreviations:
a. eCRFs: Electronic Case Report Forms
b. CDMS: Clinical Data Management System
c. pCRFs: Paper Case Report Forms
d. TPV: Third Party Vendor
e. EDC: Electronic Data Capture
f. CRA: Clinical Research Associate
g. QOL: Quality of Life
h. IRT: Interactive Response Technology
i. SAE: Serious Adverse Event
j. eCOA: Electronic Clinical Outcome Assessments
k. ROI: Return on Investment
Originally published by -
(Reimagning Clinical Trials through Digital Transformation as a Domain Consultant/ Business Analyst at Infosys)
Itís difficult to find knowledgeable people in this particular subject, however, you seem like you know what youíre talking about! Thanks
Itís difficult to find knowledgeable people in this particular subject, however, you seem like you know what youíre talking about! Thanks
I was very pleased to find this site. I wanted to thank you for your time for this particularly wonderful read!! I definitely savored every little bit of it and i also have you book-marked to see new stuff in your site.
For most recent news you have to pay a visit world wide web and on web I found this site as a most excellent web site for most up-to-date updates.
Excellent post. I was checking constantly this blog and I am impressed! Extremely useful information particularly the last part 🙂 I care for such info much. I was looking for this certain info for a very long time. Thank you and good luck.
Hi my loved one! I wish to say that this article is awesome, great written and come with approximately all important infos. I would like to peer extra posts like this .
We stumbled over here by a different page and thought I may as well check things out.
I like what I see so now i am following you. Look forward to looking at your web page yet again.
After I initially commented I seem to have clicked on the -Notify me when new
comments are added- checkbox and from now on each time a comment is added I recieve four emails with the exact same comment.
Perhaps there is a way you are able to remove me from that service?
Appreciate it!
I am often to blogging and i actually admire your content. The article has actually peaks my interest. I’m going to bookmark your site and preserve checking for brand new information.
Great – I should certainly pronounce, impressed with your web site. I had no trouble navigating through all the tabs as well as related info ended up being truly simple to do to access. I recently found what I hoped for before you know it in the least. Quite unusual. Is likely to appreciate it for those who add forums or anything, site theme . a tones way for your client to communicate. Excellent task.
I will right away seize your rss as I can’t find your email subscription hyperlink or newsletter service. Do you’ve any? Kindly allow me realize in order that I may subscribe. Thanks.
With havin so much written content do you ever run into any issues of plagorism or copyright infringement? My website has a lot of completely unique content I’ve either written myself or outsourced but it seems a lot of it is popping it up all over the internet without my agreement. Do you know any ways to help prevent content from being ripped off? I’d certainly appreciate it.
It is perfect time to make some plans for the future and it’s time to be happy.
I’ve read this post and if I could I desire to suggest
you some interesting things or tips. Perhaps you could write next articles referring to this article.
I want to read even more things about it!
certainly like your web-site but you have to check the spelling on several of your posts.
Several of them are rife with spelling issues and
I in finding it very bothersome to tell the reality then again I will definitely
come again again.